Humans have been analyzing data right from the birth of civilization. The earliest preserved records of writing are examples of data analysis. In ancient Iraq, scribes made lists of workers, creating one of the first databases in the process. They also calculated their wages directly from this raw data, a form of primitive data analysis. Data analysis is rooted in statistics, the beginnings of which can be traced to Egypt, as it took a periodic census for building the pyramids. People have taken on incredible quests and voyages, documenting their experiences along the way, helping us build a reservoir of data. In this blog, we will understand the role of data analytics in the past and how it is becoming crucial with each passing year.
“Information is the oil of the 21st century, and analytics is the combustion engine.” – Peter Sondergaard.
A few centuries ago, we lacked the necessary tools to make the most out of our data and information. Today, the availability of an excess amount of data makes it hard to extract valuable pieces of information. We have collected more data than ever in the last five years.
As per the 2018 Global State of Enterprise Analytics Report, 57% of enterprise organizations make use of big data and analytics to accelerate process and cost efficiency.
Data remains a valuable commodity when utilized properly. The need of the hour is to analyze these big chunks of data in order to extract meaningful insights and make the most out of it.
Twentieth-century: First Steps
It would be inaccurate to call data analytics a new concept. While the terminology may be new, the practice has been around for a long time, way before the age of computers! A century ago, we recorded data in good old paper files. Analysis of recorded data took place by identifying trends on statistics observed by the naked eye. Although this was better than basing decisions on intuition, it was still very flawed as there is a limit to what the eye can observe and analyze.
Pierre Simon Laplace proposed in 1820 that scientific observations had a common pattern of errors. This idea sparked a revolution in data analytics, statistics, and mathematics. As the ecosystem matured in the 20th century, statistics and mathematics were soon used to solve complex, real-life problems.
From 1962, when John W. Turkey wrote about the “Future of Data Analysis,” to 2008, when Dr. DJ Patil and Jeff Hammerbacher first coined the term “data science” the concept of data analytics has undergone a massive shift.
Then came the digital world. Methods of collecting and storing data moved from a physical setup to a cyber setup. The 20th century saw the advent of the first digital computers which were enormous in size and complexity, and were primarily used for military purposes. With the growth of computer technology and the advent of the microprocessor in the 1970s, computers became smaller and cheaper, and were used more for business applications. Data was stored on computers using magnetic tapes or disks, and the main task of business-intelligence professionals was to extract information from data stored on these devices. The development of new software technologies, such as structured query language (SQL), gave business managers the ability to access and manipulate data stored in computers. And so database and database management systems were born. With more space to store and process data, the concept of high-volume data analysis also came about rapidly. To accommodate this, we began using relational database models and spreadsheets.
The Digital Revolution
The rise of the internet and the emergence of social media brought a new era of consumer expectations. They gave birth to the disruptive nature of data, and sparked the need for data analytics.
Data analytics experts found a new way to process, understand, and utilize data to create significant business insights. This was the beginning of a new digital revolution, fueled by big data and analytics. Big data refers to the large volumes of data that are created every day. The data could be from social media posts, IoT devices, images, videos, and other unstructured data sources. The amount of data being created every day is so large that it is difficult for organizations to store the data in their computers or on their servers.The complexity of handling data has increased, along with data analysis. We understood it would become a problem down the line, so we created tools to make this process easier for business users.
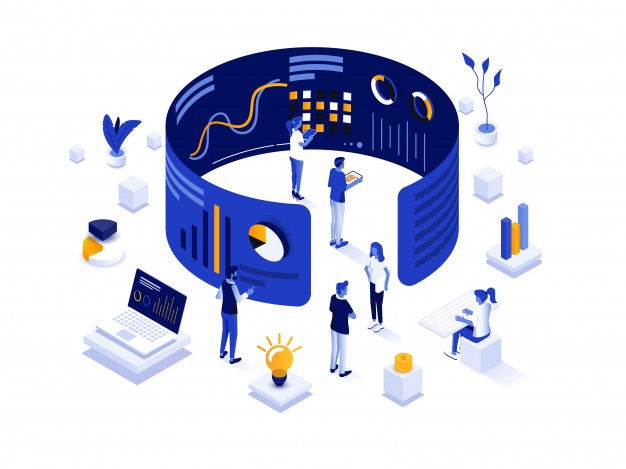
Tools such as MS Excel came with various built-in functions to make calculations and statistics easy for business users. Relational databases supported by programming languages such as MySQL and Oracle Database further allowed query data to sort, filter, and perform advanced operations on the database.
While these inventions made things much easier, their biggest drawback was that they simplified only one part of the problem – extracting statistics from the data. The analysis part of it, which involves going through the numbers to generate meaningful insights, remained a responsibility of the business user.
Thus, there was a need for automated data analysis in the cloud, and to comply with this need, we saw the rise of Artificial Intelligence and its applications, including data science.
The Age of AI
As the name suggests, data science is the science of playing with data to extract some meaning from it. We use a data science methodology to create data models that can prepare and process the data, analyze it, and generate valuable insights. We first train these data models using machine learning algorithms and then test them on a sample data set to evaluate the accuracy of their predictions. Based on the evaluations, we make additional changes and then retest the new model. The repetition of this process takes place several times to have a foolproof data model. It is then used to analyze new data and give highly accurate insights.
Currently, business analysts are deploying similar data science models to gain accurate insights. They are using it in their business to make analytical forecasts. The power of automation and AI has simplified the work of a data analyst.
Nowadays, self-service, AI-based analytics tools help analyze data from hundreds of sources to find what works and what doesn’t. Another added advantage of relying on data science is that the algorithms used in these models do a comprehensive job of analyzing the data, identifying patterns and data trends on a much deeper scale. This leaves no room for human errors that otherwise invariably creep in. This allows users to extract extremely useful bits of information that even the most skilled data analytics expert can miss.

The Rise of Business Intelligence
The business intelligence industry has grown exponentially over the last few years. Valued at 20.516 billion USD as of 2020, the business intelligence sector is predicted to grow to a whopping 40.50 billion dollars by 2026.
A business intelligence platform is an end-to-end business analytics tool that generates actionable intelligence within seconds of data loading. With business intelligence platforms such as INSIA, all you need to do is connect your data sources to the platform. Data can be in CSV, Excels, Spreadsheets, Marketing channels like FB and databases like MYSQL etc.
The BI platform performs all tasks associated with data science and data analysis by itself. It gives you ready-to-use insights in various forms. Through these BI platforms, companies can get a detailed analysis report within seconds. This allows them to make quick decisions that are factual and impactful for the overall functioning of the organization. BI tools provide a centralized platform where an organization can access its data and create reports and charts that can be easily shared with colleagues. They also let you visualize the data in the form of graphs or charts so that the information is easier to understand.
Future prospects
Data analytics has now become a necessity for businesses. The evolution of AI and machine learning, the Internet, and other technological advances have created new ways to process and analyze data. With big data, the need for real-time analytics has increased. Now, organizations must be able to process the data as it is being created and understand the insights from it. There is an increased focus on integrating data from different sources into one platform to create actionable insights. Data visualization and artificial intelligence are being used to create interactive data visualizations that are easier to understand. We are seeing a global effort to simplify the process of generating insights from data. A few features that are entering the Business Intelligence sector and are helping in realizing this vision are:
Search bar:
When we talk about getting information from the internet, the first thing that comes to our mind is search engines. All it takes is to open Google and type what you are looking for. Companies like INSIA have introduced search engine-like functionality that accepts keywords in natural language. and the platform will automatically show relevant insights .
Personalized dashboards:
Dashboards enable business users to visualize their business metrics’ performance. INSIA provides users with a well-described business analysis report through personalized dashboards. These dashboards often include tables and graphs of metrics and KPIs that you need to focus on. It also accompanies various insights that help you make critical business decisions. Users can personalize the dashboard as per the business needs, to make information highly relevant and easy to understand.
Push automated insights:
Nowadays, applications give us updates by sending push notifications to our phones and emails. BI platforms are replicating this to provide business users with automated insights tailored for them.
This saves a lot of time as it maintains a live track of data with real-time analysis, giving users performance updates as they occur.
Final Notes
History is proof that the way we analyze data has reformed the business outlook for organizations. Companies that adopted new methods to increase volume, speed, and efficiency of data analysis have gained a competitive advantage and have remained relevant.
Being in business is not simple.
Today, it has become necessary to keep one’s business up to date with the latest data analytics tools to survive and soar high in the business world.